How AI and ingenious apps are changing the face of medical research
For just a small sample of your saliva and around $100, there are services that will analyse your DNA at more than 700,000 genetic markers. In six to eight weeks, you can find out if you’re 23% Polynesian or 6% Viking, among many other unique characteristics.
It’s reasonable to think that if our genes can tell us where we’ve come from, they can also tell us where we’re headed, especially with regards to our health.
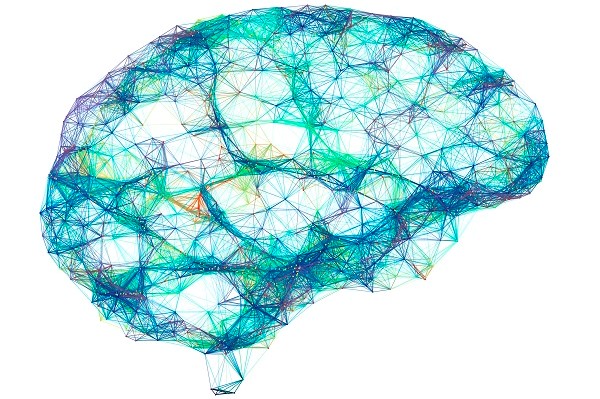
“There’s a sense in which people think precision medicine is all about the gene,” says Professor Enrico Coiera, Director of the Centre for Health Informatics at the Australian Institute of Health Innovation at Macquarie University.
But we are far more than being simply the sum of our genes.
We are, in fact, our ‘phenome’: the sum of all the ways the environment we live in has influenced the expression of our unique DNA. This interaction between genes and the environment creates observable traits, called phenotypes.
Our health records are full of information on the phenotypes we display. Do we struggle with our weight? Are we at risk of a chronic disease like diabetes? Do we respond poorly to a particular medication or a combination of medications?
The increasing use of electronic health records means clinicians and medical researchers are sitting on a gold mine of data.
This could be used to target precision healthcare in the years to come, with the help of artificial intelligence (AI) and a sub-discipline of AI called machine learning, which uses algorithms to ‘learn’ from large volumes of data in order to make a determination or prediction.
“We can use statistics and machine learning to try and work out what the right thing is for you, even in the absence of strong trial data,” Coiera explains.
Intelligent machines
Clinical trials, by definition, only compare the effectiveness of treatments in people with one disease, and only a small proportion of conditions have clinical trial data available to them. Yet clinicians regularly need to recommend treatments for patients with a complex mix of health issues.
In the future, clinicians will be able to use AI to examine the success of treatments in a virtual cohort of people who are very similar to you – same age, same gender, comparable disease history – to recommend a treatment that best matches your phenome.
Machine learning algorithms will predict various outcomes for patients and help guide the decisions of GPs that extend far beyond the simple risk-prediction calculators that they currently use.
“The idea behind machine learning approaches is that it’s not just a static algorithm,” explains Professor Louisa Jorm, Foundation Director of the Centre for Big Data Research in Health at UNSW. “It continues to learn as more and more patient information comes in.”
As with any new technology, the benefit of using clinical AI systems is not without risk. One of the potential problems is automation bias. “This is the human tendency to believe whatever the machine tells them to do,” says Coiera.
It’s important that doctors don’t view the algorithms as some sort of ‘black box’ and that they still exercise personal judgement around their recommendations to patients, Jorm adds.
Social network disease
Researchers are discovering that, for certain health issues, rather than being inherited through our genes or determined by our postcode, they are spread through our social networks.
“We are social animals, so we’re shaped by what others do,” says Coiera.
Our social ties are no longer primarily determined by geography. Our ideas and behaviours are increasingly shaped by what people in our social media network post, comment on, and like.
Researchers from the Centre for Health Informatics used machine learning methods to analyse how exposure to information about the human papillomavirus (HPV) vaccine on Twitter influenced the take-up of the vaccine across the US.
They analysed more than 270 million exposures to 258,418 tweets posted over two years, by teaching machine learning algorithms to differentiate between pro- and anti-vaccine sentiment.
They found that vaccine coverage was lower in states where safety concerns, misinformation, and conspiracies made up a higher proportion of exposures. This type of analysis helps population health experts create location-specific strategies to overcome the spread of misinformation through social networks.
Concerns over the number of people live-broadcasting their suicide attempts on Chinese social media recently led researchers, headed by former Black Dog Institute research fellow, Dr Ang Li, to do some statistical modelling. Her team looked at the language patterns of people who responded to suicide posts on the Chinese microblogging platform, Weibo.
As they reported in 2018 in the journal Asia-Pacific Psychiatry, they found that more than one-third of people expressed attitudes that stigmatised suicide, and that these stigmatising attitudes were strongly related to a poor understanding of mental health and suicide.
The language classification model developed as part of the study can now be used to detect suicide warning signs and develop targeted suicide prevention messages for use on social media.
Gaming the answers
Harnessing the possibilities of big data in medical research is not simply the preserve of machines.
The sheer volume and variety of big data, and the speed at which it’s being created, means help is now being sought from ‘citizen scientists’ to solve some of the most difficult problems in medical research.
Foldit is harnessing the competitive spirit and puzzle-solving ability of online gamers to predict the structure of the proteins that are central to the function of diseases such as Alzheimer’s, HIV, and cancer.
A protein is made up of a chain of hundreds of amino acids that consistently fold into a specific shape. Understanding how proteins fold into shape will help researchers design new proteins to combat the disease-related proteins.
Those of us with little free time to spare can also contribute to medical research, even while we’re sleeping. The DreamLab app, a collaboration between the Garvan Institute of Medical Research and the Vodafone Foundation, uses the processing power of thousands of idle smartphones to compute millions of research calculations every night of the week.
More than 122,000 DreamLab users have helped the Garvan Institute complete a data analysis for Project Decode, which aims to understand cancer based on patients’ DNA profiles. This halved the time it would have taken using the Institute’s computing infrastructure alone. Another DreamLab project, Project Genetic Profile, is ongoing.
Taken together, the combination of DNA testing, personalised medicine, the application of big data to healthcare, new computational methods to analyse detailed health records, and the clever use of smartphone apps, means we could see IT bringing big improvements to our health now and in the coming decades.
Bring on the future.
By Viki Cramer
Updated 2 years ago